How would a project that combines the hottest technological concepts of AI and the popular blockchain sector, DePIN? Today, let’s discuss such a project that inherently carries topics and buzz—io.net.
io.net is a decentralized GPU network built on Solana designed to give unlimited computing power to ML/AI applications. In March this year, io.net raised $30 million in a Series A funding round, bringing its total funding to $40 million, including last year’s seed round, with a latest valuation of $1 billion. Investors include Hack VC (Series A lead), Multicoin Capital, Delphi Digital, Foresight Ventures, Animoca Brands, Continue Capital, Solana Ventures, Aptos, LongHash Ventures, OKX Ventures, Amber Group, SevenX Ventures, and ArkStream Capital, etc.
Computing: The Most Successful Business Model of DePIN
Messari’s 2024 report mentions that the DePIN is flourishing recent years. As of the end of 2023, the DePIN ecosystem had launched over 650 projects, with a total market value exceeding $20 billion and an annualized on-chain revenue of $15 million. Messari classifies DePIN into six categories: compute, wireless, energy, artificial intelligence, services, and sensors. The number of projects, market cap, and annualized on-chain revenue for each category are as follows:
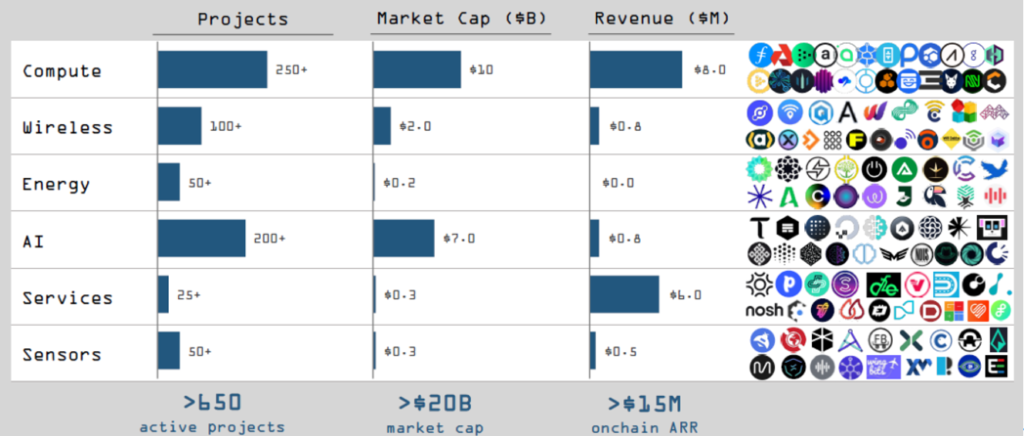
resource:https://messari.io/report/state-of-depin-2023
As shown in the chart, compute can be considered the most successful business model in terms of the number of projects, market size, and revenue. The current strong demand for compute power from ML and AI (according to an OpenAI blog post, the amount of computing needed to achieve state-of-the-art machine learning results doubles approximately every 3.4 months, roughly 40 times every 18 months) has made this field more critical than ever. Additionally, according to io.net’s research on official website, the current shortage in cloud GPU capacity is likely in the range of 5-10 exaFLOPS. In the near future, cloud GPU capacity must expand 2-3 times current levels in order to meet user demand.
io.net: Decentralized GPU Cluster
io.net increases GPU computing power by accessing underutilized GPU sources outside of the cloud such as independent data centers, Crypto miners, and crypto projects such as Filecoin or Render, which together will unlock fair access to computing power by assembling 1 million + GPUs. The following screenshot from io.net’s website shows the computing power at the time of capture:

https://explorer.io.net/explorer/home (25 July 2024 18:07 GMT+9)
Ideally, by accessing current resources, io.net can provide an additional 200 exaFLOPs of GPU capacity.
Compared to other projects which also work for the DePIN compute, io.net focuses more on GPU computing resources. According to current data, the scale of its GPU network is already over 100 times larger than similar projects, and its prices are generally 10-20% of traditional centralized cloud services, making it highly competitive. By the adoption of blockchain’s decentralized technology, io.net can make the entire service process more transparent, traceable, and significantly more secure. Additionally, io.net integrates Solana’s payment system and issues IO tokens to provide attractive ignition incentives for resource providers, which is the basic characteristic of blockchain projects—the token economy for the building of the project ecosystem.
Four Core Functions of io.net
Batch Inference and Model Serving
io.net allows machine learning teams to build out inference and model-serving workflows across a distributed network of GPUs. io.net is natively built on top of ray.io, and support all the frameworks that ML engineers use for workload distribution, including Anyscale, Pytorch FSDP, TensorFlow, and Predibase. Custom cluster creation is also supported, allowing for selections and options such as clean energy, geographic location, security compliance level, connectivity tier, and cluster purpose.
And performing inference on incoming batches of data can be parallelized by exporting the architecture and weights of a trained model to the shared object-store.
Parallel Training
CPU/GPU memory limitations and sequential processing workflows present a massive bottleneck when training models on a single device. io.net, with its decentralized and inherently distributed model, leverages distributed computing libraries to orchestrate and batch-train jobs such that they can be parallelized across many distributed devices using data and model parallelism.
Parallel hyperparameter tuning
The quality of a model often depends on various hyperparameters, such as the number of layers, hidden units, and batch size. To find the best model, developers must search through different hyperparameter settings, and this process can be quite resource-intensive.
Hyperparameter tuning experiments are inherently parallel, and io.net leverages distributed computing libraries with advanced Hyperparam tuning for checkpointing the best result, optimizing scheduling, and specifying search patterns simply.
Reinforcement learning
Reinforcement learning algorithms involve a large number of simulations. io.net uses an open-source reinforcement learning library, which supports production-level, highly distributed RL workloads alongside a simple set of APIs, including Public API for the website, Private APIs for Workers/GPU Providers and Customers, and Internal APIs for Cluster Management, Analytics, and Monitoring/Reporting.
Summary
Due to the rigid demand for computing resources driven by the rapid development of the AI/ML industry, investment institutions hold a positive attitude towards solutions in the computing sector. Unlike the projects we discussed before whose mainnets have not yet launched, io.net’s services are already available. However, it seems that most of their collaborations are still within the blockchain industry, and community building has most relied on airdrops. For such technical projects, Paralism hopes to see more substantial business progress.
However, if io.net wants genuinely collaborate with Web2 projects, the efficiency of settlement will inevitably be affected by the performance of the blockchain itself. This will be one of the bottlenecks preventing large-scale application of DePIN projects. Many projects have made relentless efforts in performance scaling, with parallel architecture being one feasible solution that Paralism believes can support large-scale applications. This has always been the core business of Paralism. Under Paralism’s parallel architecture, DePIN projects can quickly issue Appchains or protocol chains, sharing Paralism’s higher TPS and execution security.
Therefore, gaining recognition from traditional industries may require more effort from io.net, both technically and in marketing.
Paralism hopes to see io.net become more pragmatic, realizing their vision by truly aggregating idle GPU resources to provide the computing power needed for AI/ML.
Paralism hopes to see io.net become more pragmatic, realizing its vision by truly aggregating underutilized GPU resources to provide the computing power needed for AI/ML.
References:https://io.net/